Nudged off a cliff
A recent meta-analysis looked like good news for the effectiveness of "nudge" theory. Does a new set of rebuttal letters throw the whole idea into doubt?
I’ve always thought “nudge” was a very unfortunate term. The idea is supposed to be that, with “nudges”, you can change small aspects of people’s environment to subtly influence the choices they make, without actually coercing them to do what you want. Some well-known examples are:
Adding questions about becoming an organ donor to forms people would otherwise be filling in, such as when they apply for their driving licence. This gets the questions—which they probably wouldn’t seek out deliberately—under people’s noses, and makes it more likely they’ll opt in to becoming a donor;
Enrolling people in pension schemes by default - they can easily opt out if they’d like, but since most people go along with the status quo, you get a lot more people saving for retirement;
Making healthy food more visible in cafés and shops, so people are more likely to pick it up;
Sending reminder emails about donating to charity;
Putting a little picture of a fly in the centre of urinals, so men target it and are less likely to “miss”, preventing them from making a mess of your bathroom floor.
These kinds of nudges—the name, of course, comes from Richard Thaler and Case Sunstein’s massively-bestselling 2008 book Nudge—are usually supported by some kind of experimental research, bringing the sought-after “evidence-based” perspective to marketing and policy-making. And the nice thing about them is that, in most cases, they’re not paternalistic or controlling - they can be used without passing new laws or imposing anything on the unsuspecting public.
But - the name. To me, “nudge” brings to mind sharp elbows - pushy and insistent people (or governments) shoving you around and intruding on your life. It also makes me think of a certain kind of misguided psychologist (or “behavioural scientist” as they always call themselves in this context for some reason) smugly insisting that they know just the clever tweak that will make the little people do X or Y desirable behaviour. As I say, it’s unfortunate, because nudges are often non-paternal and even quite libertarian.
Alas, the more formal, scientific-sounding name for nudges—“choice architecture interventions”—if anything sounds even worse, invoking one of those business seminars where everyone talks in pure management-speak about actioning synergy to leverage stakeholder competencies going forward, or whatever.
Anyway - unfortunate as it is, the name doesn’t really matter. What matters is whether nudges work. And at the very start of 2022, we seemed to have found a resounding answer.
The meta-analysis
“Nudges prove their effectiveness”. That was the headline of the press release that appeared alongside a new meta-analysis, in mid-January this year. The meta-analysis, which included over 200 studies with nearly 450 experiments, involving more than 2 million participants, was published in the Proceedings of the National Academy of Sciences (PNAS for short - no jokes please). Here’s how the authors, psychology researchers from the University of Geneva, described it:
[W]e quantitatively review over a decade of research, showing that choice architecture interventions successfully promote behavior change across key behavioral domains, populations, and locations.
The authors dug into the data in more detail, showing that “decision structure” nudges (ones where defaults are changed, or the range of options are grouped differently, like the pension one above) had a stronger effect than “decision information” ones (where relevant information is highlighted or otherwise made more visible, like the organ donor, food, and urinal-fly ones above) or “decision assistance” ones (e.g. giving people reminders, like the charity one above). They also found that nudges had the strongest effects on food-related decisions, and smaller effects on finance-based ones.
As soon as the study appeared, though, it hit some pretty dangerous rocks. The researchers had included in their analysis one study that had been retracted for being fraudulent (it was the 2012 study co-authored by Dan Ariely, which you might remember being shown to be fraudulent about a year ago - still nobody knows who committed the fraud…!). It was a sad illustration of how fraudulent research can lie in wait—in this case for a whole decade—before tainting the results of future review studies.
That fraudulent study had been retracted. But what about work that’s unretracted, but still under a cloud of suspicion? You might remember that I told the story of Brian Wansink in my book Science Fictions. He’s the disgraced Cornell University food-psychology researcher who inadvertently admitted to fiddling his data in a now-infamous blog post, went on to have more than a dozen papers retracted and many more corrected, and lost his job as a professor. I counted eleven papers in the nudge meta-analysis (you can see the list in their Supplementary Materials) that were first-authored or co-authored by Wansink. They’re among the ones that haven’t formally been retracted - but do you trust this guy to have produced reliable research, even in the studies that weren’t so dreadful they had to be expunged from the scientific literature?
In a correction they posted in May, the meta-analysis authors noted that they’d removed the retracted Ariely study from their analysis and re-run the stats - but didn’t do anything about all the Wansink papers. At the least it would be interesting to see what would happen to those very strong results for food outcomes if Wansink’s papers were removed (though since they’ve shared their data online—and good on them for doing so—anyone could do the sans-Wansink re-analysis if they wanted).
In any case, for now let’s try to forget that these studies were included - it’s only eleven out of several hundred, after all. After the removal of that retracted study, the effect size of the meta-analysis didn’t change much. And it was still a pretty impressive number: the average effect of nudges, across all experiments and contexts, was a difference of 0.43 standard deviations (SDs; for stats fans, a Cohen’s d of 0.43) between the nudged group and the control group. In the common statistical rule of thumb, this would be above a “small” effect (0.2 SDs) and approaching a “medium” one (0.5 SDs). In the context of behavioural interventions, or even many medical treatments, though, it’s substantial: an equivalent would be an intervention that boosted someone’s IQ (SD = 15 points) by six and a half points, for instance (or their height—SD ≈ 6cm—by about 2.5cm).
The meta-analysts write that:
…while the majority of choice architecture interventions will successfully promote the desired behavior change with a small to large effect size, ∼15% of interventions are likely to backfire, i.e., reduce or even reverse the desired behavior, with a small to medium effect[.]
The backfire effect is quite amusing, and immediately makes me think of men deliberately avoiding the little fly in the urinal just out of spite. But some of this backfire effect is probably just noise: even if an effect is real, we wouldn’t expect every study to find it, just because of sampling variation - and we’d even expect the odd study to find the opposite effect sometimes.
But in any situation where you have a vast majority of positive effects (85%, as per the quotation above), it should make you wonder: are we seeing the full picture here? Which brings me to the very important issue of publication bias. I recently discussed this in the context of studies of breastfeeding and intelligence: scientists, peer-reviewers, and journal editors are more likely to speed a study on its way to publication if it has a positive result, because positive results are usually good-news stories (the intervention works! We can change people’s behaviour!). But since this means that null (or negative) results—results which are, presumably, just as real as the positive ones—are less likely to get published, we end up with a distorted view of the research.
And the publication bias on nudges is wild.
Putting the “fun” in “funnel plot”
Below is the funnel plot from the meta-analysis. It plots the effect size of the study (the Cohen’s d that I mentioned above) on the x-axis and the precision of the study (basically a measure of how big, in terms of sample size, the study is; it’s measured by the standard error) on the y-axis. Because we’d expect the bigger, more precise studies to be more accurate on average, we expect them to produce a better estimate of the true mean effect. Smaller studies will vary more and more as they get smaller and smaller, sometimes producing a spuriously big effect, sometimes a spuriously small one, just through chance. So the dots, one per experiment, should fan out into an upside-down funnel shape on the plot (the outline of which is helpfully provided in white here):
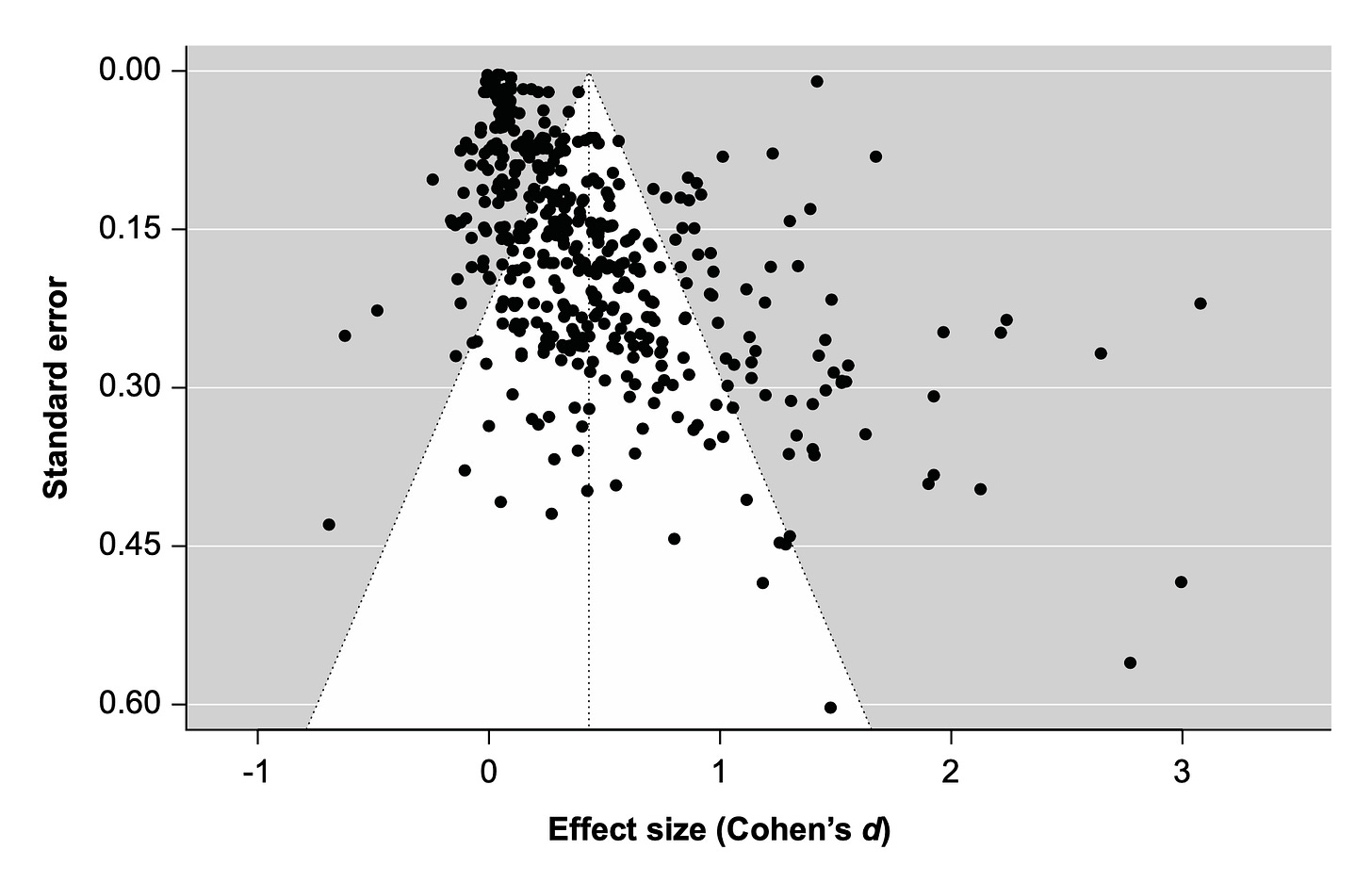
The dots here, to put it mildly, don’t fan out into a funnel shape. There are very many on the right side of the funnel, but very few on the left, and while they do generally get more disparate as you get lower on the x-axis (smaller studies producing more variable results), the distribution is far from symmetrical.
Here’s another way of looking at the same data, from a letter that was published in PNAS just the other week in response to the meta-analysis. This is called a “raincloud plot”, hopefully for obvious reasons; the little “clouds” show the distribution of the studies in each category. It splits the studies up into their behavioural categories (food, finance, etc.):
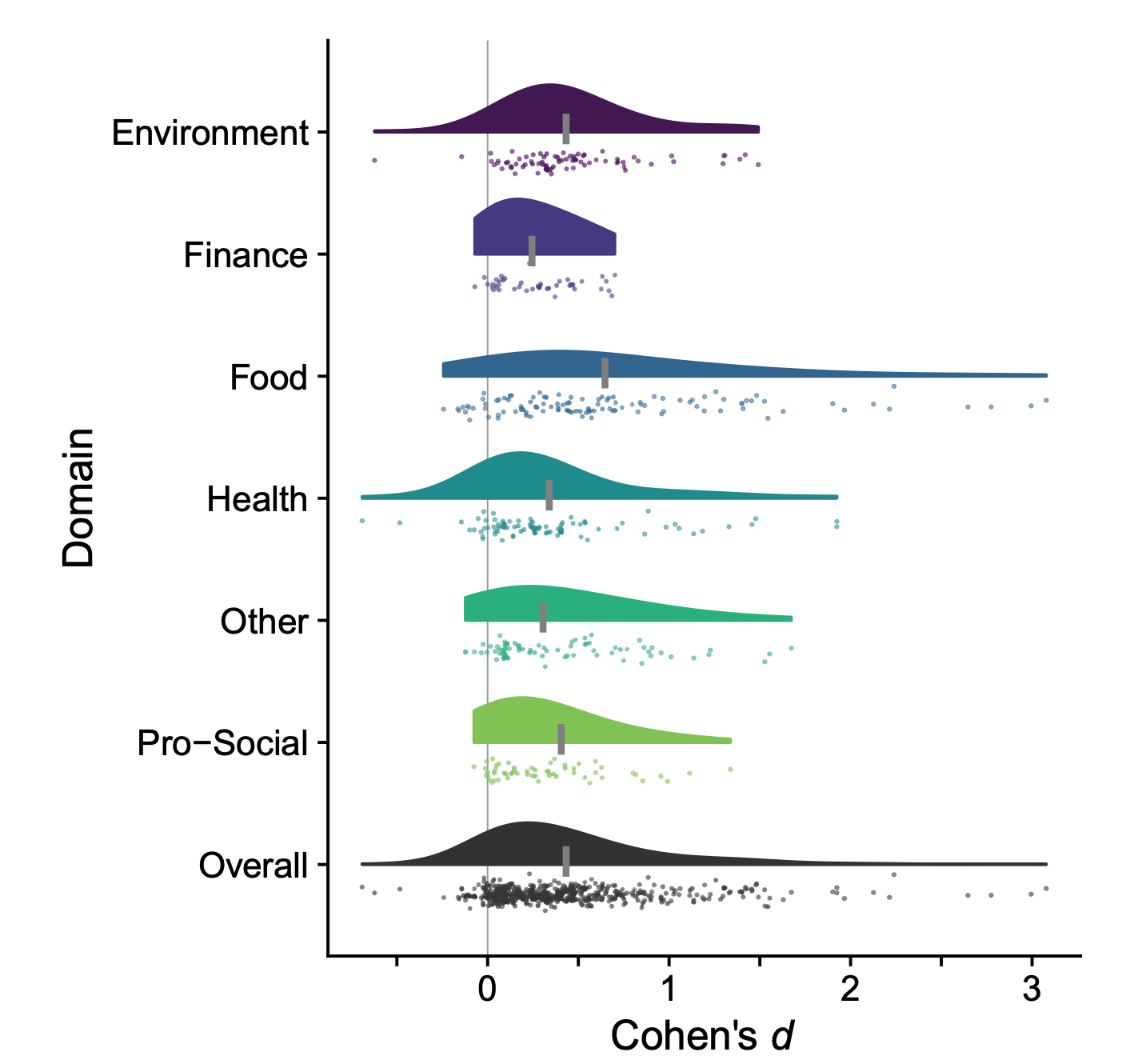
Note what you see for almost every category: a big long tail to the right (where there are a few studies with very big positive effects) and, to quote the letter-writers, a “left-truncated” distribution, where there’s nothing approaching the same long tail on the left-hand side. There barely seem to be any studies that show very big negative effects, even though—as noted above—we’d expect to see a lot of them by chance.
To put it another way, in a perfect world we’d want these distributions to be something approaching symmetrical, just like we’d want the nice symmetrical funnel shape in the funnel plot. Since we don’t have that, it seems like something is missing. The makers of the raincloud plots note in their letter that:
A plausible mechanism for this left “cliff” [on the raincloud plots] is suppression of unfavorable results.
In other words: the reason the plots look like that is because scientists don’t bother publishing—or are substantially less likely to publish—nudge studies that don’t find positive effects: it’s publication bias.
The original meta-analysts had noticed this possibility. They ran some calculations using “Egger’s test”, a commonly-used way to check for publication bias that’s based on the funnel plot. They came up with some estimates of what their effect size would look like if the publication bias was bad, or really bad. If it was moderate, the effect would drop from d = .43 to .31. But if it was severe, the effect would drop all the way to d = 0.08 - perhaps still interesting (it translates to 0.48cm in height, or 1.2 IQ points), but a far cry from the chunky effect size that was the headline finding of their meta-analysis.
Another response letter, also newly published in PNAS, takes things a step further. First, it notes that, aside from a few brief mentions, the publication bias analysis wasn’t really integrated into the meta-anaysis as a whole - indeed, the meta-analysts “proceed largely without taking publication bias into account in their subsequent analyses”, and it didn’t seem to affect their overall conclusions (or, I might add, their press release). Second, the letter-writers argue that the publication bias, as revealed by the funnel plot and the associated analysis was, in fact, severe. Third, they run their own publication bias correction.
This was based on a newly-developed statistical technique for meta-analysis called “Robust Bayesian Meta-Analysis”, or RoBMA for short. As with all Bayesian techniques, this can give us evidence for the null hypothesis, which is something normal methods—based on p-values—can’t do. And it can do so after having corrected for publication bias in a meta-analysis (you can read about the maths, and see some examples of RoBMA being used, in a new paper). Using RoBMA, the letter-writers found that not only was there strong evidence for really bad publication bias, and not only did correcting for this reduce the effects to near-zero in almost every case, but that there was, for at least some of the categories, strong evidence that there was no overall effect of nudges in the literature. In other words, their correction pushed the effect of nudges entirely off a cliff. They concluded:
[O]ur Bayesian analysis indicates that, after correcting for this [publication] bias, no evidence remains that nudges are effective as tools for behaviour change.
Ouch! Is there anything that can save the idea of nudging from such a drastic conclusion?
It turns out: yes. Sort of.
Baby/bathwater
There was a third rebuttal letter to the PNAS meta-analysis. This one mentioned publication bias too, but the main point it made was about variability. Remember how the meta-analysts said that nudges “successfully promote behavior change across key behavioral domains, populations, and locations”? This, the third set of letter-writers argued, is highly implausible - the idea that you can take one number (d = 0.43!) for an effect size across all the different nudge types, behavioural outcomes, contexts, countries, and so on, and say it’s “the average effect of nudges” is, when you think about it, a little absurd. Even just the five nudges I listed at the top of this post are all totally different - what’s the “average effect” of the urinal intervention and the one about signing up to a pension scheme?
To be fair to the meta-analysts—who, by the way, wrote a timid response to the three critical letters where they basically just agree with all the criticisms—this is a problem with meta-analysis in general: even though there are a whole bunch of ways to explore the heterogeneity in your dataset, there’s still a feeling that, when you’re summarising your meta-analysis as a whole, you should give that one number that sums everything up - even if that’s a ridiculous oversimplification of your dataset. The letter-writers argue that, given the high degree of variability:
with a few exceptions (e.g., defaults), we see no reason to expect large and consistent effects when designing nudge experiments or running interventions.
And those expectations are important. Researchers who are planning a study use the previous literature—especially authoritative studies like meta-analyses—to work out how many participants they think they’ll need. If they’re expecting big effects, they’ll do a smaller study. But if that expected effect has spuriously been inflated by publication bias, they’ll end up doing too small a study - and the cycle of low-quality research will continue.
Just a second! In that quotation above, did they say “with a few exceptions”? Maybe there’s something salvageable here! Indeed, even the RoBMA letter-writers noted that:
…all intervention categories and domains apart from “finance” show evidence for heterogeneity, which implies that some nudges might be effective, even when there is evidence against the mean effect.
It’s kind of obvious, I suppose: if you chuck a whole array of widely-varying effects into a meta-analysis, it could be the case that some work even if your overall (bias-adjusted) result is null. For nudges, it does seem plausible that the “default” ones, like defaulting people to opt in to a pension scheme, would have an effect (though a 2019 meta-analysis of just the studies on defaults showed a lot of variability even just within this category).
This is really all another way of asking: “what was the point of this meta-analysis?”. It was an exercise in apples-to-oranges comparison that just leaves everyone more confused than when they started. Instead, what we’d want is a series of meta-analyses of close replication studies of similar nudges, so the between-study heterogeneity is kept low and we can really interpret the effect size numbers.
But for now, getting an idea of these effects means dredging through a messy, confusing, and biased academic literature. Depressingly, if there are any other reliable places to look for evidence, it’s often better to look there than in scientific journals, which are subject to the terrible publication bias problems we’ve seen above. And interestingly, there’s another recent review study that does just that. They took a sample of nudge papers published in the academic literature and compared them to all the randomised nudge trials done by two “Nudge Units” in the US. Some of these trials were published in journals, but to these reseachers, there was no such thing as publication bias: they had details on every experiment the Nudge Units had ever run.
They found two things in the academic, journal-published studies: a really big effect on average (they expressed it in terms of the percentage difference between the treatment and control groups: 8.7%), and the usual telltale signs of publication bias. The big effect, in other words, could mainly be an illusion. In the Nudge Unit samples, though, things were different. First, the research was higher-quality, including much bigger sample sizes and more transparent research designs. Second, there was a substantially lower effect size: a difference of only 1.4%.
The conclusion we should draw from this paper—which is long, but very worth a look—is that some nudges do seem to have a real impact, albeit a much smaller one than we’ve been led to believe. And that makes sense! It’s really hard to find big, or even moderate, effects in this kind of area, and the d = 0.43 number should’ve given the meta-analysts (and their peer-reviewers, and their editor) some serious pause. It could only have come about from applying the blunt instrument of an overall meta-analysis to an academic literature—full of publication bias and low-quality research and sometimes even scientific fraud—that’s badly screwed up.
There’s a massive set of published studies on nudges, and there’s often considerable excitement in the media (egged on by the researchers’ press releases) when a new paper comes out. Inadvertently, by hinting at how serious the effect of publication bias could be, the PNAS meta-analysis puts that whole literature under a cloud. Until academic nudge researchers dramatically up their game—we’re talking Registered Reports, replication studies, open data, open code, the whole thing—we should be extremely sceptical of anyone who leans on this literature to suggest any policy changes.
Perhaps the name “nudge” is unfortunate for another reason. Maybe by using this one catchy name for the whole varied range of interventions to change people’s behaviour, we’ve papered over some really big differences between them. We’ve encouraged meta-analysts to think that they can go out and find “the effect” of nudging. And we’ve allowed the halo effect from some nudges that really do work to add some unjustified lustre to those that don’t.
It might be that the best way to get rigorous about nudges is to stop talking about “nudges”. And wouldn’t that be a relief?
Image credits: Opening image from Getty. Funnel plot from Mertens et al. (2021) - shared under a CC-BY licence. Raincloud plot from Bakdash & Marusich (2022) - shared under a CC-BY licence.
I'm sorry to bother you with this as I am sure you must be thinking about it, but what do you think of the recent review which claimed that serotonin has no relationship to depression? Would love to read your take on it.
(I sincerely hope) there’s a special place in hell for government bureaucrats and nudge-ists who think “changing the default” is a benign “nudge”.
Making something opt-out rather than opt-in is not some brilliant science-based choice architecture hack.
It’s simply coercing users by taking away their freedom to choose.
Compare “changing the default” to other judges and it’s clear that this is the most coercive technique (yet).
It is therefore no surprise that this is mentioned as one of the more effective methods.
Sorry for the rant but I had to get this off my chest.
Thank you for this essay/post (sorry, no idea what substack posts should be called), I really enjoy your writing